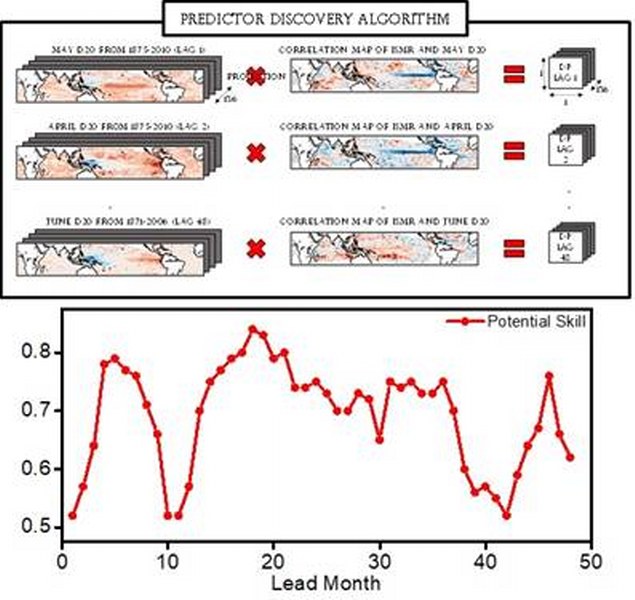
Predictor Discovery Algorithm shows AI can improve predictability of Indian Summer Monsoons
New Delhi: A newly devised algorithm powered by Artificial Intelligence can help increase the predictability of the Indian Summer Monsoons (ISMR) 18 months ahead of the season.
The algorithm called predictor discovery algorithm (PDA) made using a single ocean-related variable could facilitate skillful forecast of the ISMR in time for making effective agricultural and other economic plans for the country, said the Ministry of Science & Technology.
While researchers have well established the scientific basis for ISMR predictability and made significant advances over the past century in understanding the variability and predictability of ISMR, the skillful prediction of ISMR even one month in advance has remained a major challenge.
Neither the potential (theoretically possible) skill (correlation between the predicted and observed ISMR) and the actual skill of ISMR forecast are available at longer lead times--6, 12, 18, 24- months ahead of the season.
Traditionally, researchers select a predictor of ISMR based on the maximum correlation of an atmospheric or oceanic variable with ISMR over a region of the globe.
Such technique restricts in the realization of the true potential predictability of ISMR as it accounts for one predictor over a particular region at a time.
Scientists at the Institute of Advanced Study in Science and Technology (IASST), Guwahati, an autonomous institute of DST along with their collaborators have found that the widely used sea surface temperature (SST) is inadequate for calculation of long-lead prediction of ISMR.
This, they found was because the potential skill of ISMR estimated by the predictor discovery algorithm (PDA) using SST-based predictors was low at all the lead months.
The team consisting of IASST Indian Institute of Tropical Meteorology (IITM), Pune, and Cotton University, Guwahati, devised a predictor discovery algorithm (PDA) that generates predictor at any lead month by projecting the ocean thermocline depth (D20) over the entire tropical belt between 1871 and 2010 onto the correlation map between ISMR and D20 over the same period.
This encapsulated all the potential drivers over the entire tropical region in order to realize the true potential skill of ISMR prediction at any lead month.
This is because this one predictor embedded the simultaneous contribution of all the potential drivers over the entire tropical belt at any lead month identified by the correlation map. Besides, the oceanic thermocline depth (D20) is least influenced by the stochastic atmospheric noise.
The new algorithm indicates that the potential skill of ISMR is maximum (0.87, highest being 1.0) 18-months before the ISMR season. At any lead month, the predictability of the annual variability of ISMR depends on the degree of regularities in the annual variability of its drivers.
With the newly discovered basis of long-lead ISMR predictability in place, Devabrat Sharma (IASST), Dr. Santu Das (IASST), Dr. Subodh K. Saha (IITM), and Prof. B. N. Goswami (Cotton University) were able to make 18-months lead forecast of ISMR between 1980 to 2011 with an actual skill of 0.65 using a machine learning based ISMR prediction model.
The success of the model was based on the ability of artificial intelligence (AI) to learn the relationship between ISMR and tropical thermocline patterns from 150 years of simulations by 45 physical climate models and transferring that learning to actual observations between 1871 and 1974.
As the potential skill of ISMR at 18-months lead is 0.87, there is still considerable scope in improving the model.
The findings published in Quarterly Journal of the Royal Meteorological Society paves way for long-lead skilful prediction of ISMR in coming years with accelerating improvement of coupled climate models together with the advent of nonlinear machine learning tools.
Skillful long-lead forecasts of ISMR like one year ahead of the season will be highly beneficial to the policymakers and farmers in planning and making the country’s food production resilient to increasing vagaries of ISMR with global warming.
Support Our Journalism
We cannot do without you.. your contribution supports unbiased journalism
IBNS is not driven by any ism- not wokeism, not racism, not skewed secularism, not hyper right-wing or left liberal ideals, nor by any hardline religious beliefs or hyper nationalism. We want to serve you good old objective news, as they are. We do not judge or preach. We let people decide for themselves. We only try to present factual and well-sourced news.